Despite being essential in organizations across sectors, data scientists wrestle with the implications of writing the code that will replace their own jobs.
Coal miners will disappear in a clean energy revolution. Taxi dispatchers are being replaced by ride sharing apps. And video rental store employees lost their jobs to Netflix years ago.
History is replete with new technologies that have rendered entire occupations obsolete, turning a lost job into much more than a lost paycheque. Beyond earning a living, our careers weave into how we define ourselves as people—when an entire occupation disappears, pieces of professional and personal identity go with it.
For data scientists, this prospect creates an existential crisis. Unlike a taxi dispatcher blindsided by the rapid rise of Uber, data scientists spend their days in high demand, busy writing the code that will automate their own jobs out of existence.
“Data scientists are actually contributing to their own demise—and they are very aware of it,” says Emmanuelle Vaast, Professor of Information Systems and Associate Dean of Research at McGill University’s Desautels Faculty of Management.
“When data scientists develop Artificial Intelligence systems, they are creating programs that can conduct analysis in other contexts,” Vaast adds. “They are working to make themselves obsolete. This is something pretty unusual.”
Evolving codes of conduct
To better understand how data scientists navigate the delicate balancing act of working with digital technologies that both enable and threaten their own occupation, Vaast and Desautels Professor of Information Systems Alain Pinsonneault analyzed six years of recent blog posts and comments in an online community of data scientists.
In addition to this tension between the occupation’s persistence and its eventual obsolescence, Vaast and Pinsonneault also explored the ways that data scientists understood their occupational identity through its similarities and differences with other jobs.
“Many data scientists think about the ways that they are different from other occupations, and this has changed over time,” Vaast explains. When Vaast and Pinsonneault began their six-year observation period, data scientists defined themselves by distinguishing themselves from statisticians: statisticians use methods that have been used for centuries, while data scientists use sophisticated programming languages such as R or Python.
As time went on, statisticians faded into the background, and other professions became more relevant to data scientists’ occupational identity, in particular software developers. Like data scientists, software developers also actively work to eliminate their positions, developing software that has the potential to take over their roles.
Occupations on the brink
Call this phenomenon persistent extinction or continuous obsolescence, by any neologism it encompasses the liminal time when an occupation will eventually become obsolete, but for the moment continues to fill a need.
The tech sector makes for concise case studies of people who work closely with the technologies that will replace them, but Vaast believes persistent extinction also has implications beyond that sector.
“Similar processes can be observed in other occupations. In Japan, robots now assist teachers, and studies of it have shown something incredible,” Vaast points out. “A lot of young children react very well to the robots because they have cute features, and they are endlessly patient. For now, they are only assisting human teachers, but at some point, this might change.”
Digital identity transformation
Data has been compared to gold and oil, but it could also be likened to air. It is vital, generated at countless diffuse points, and so ubiquitous that people often forget it’s even there.
In the 21st century, working with data plays into many different professions, from social media manager to quantitative investment analyst to software programmer. “People in many other occupations use the same digital technologies, so that creates a pattern in which different occupations become closer to one another,” says Vaast.
We need to think about this ongoing transformation as a part of occupational identity.
That ubiquity of data-driven technologies and related roles makes it tricky to answer the question of what, exactly, makes a data scientist? Generally, data scientists are distinguished by their advanced data skills and fluency in programming languages that enable them to generate insights from large data sets and qualitative unstructured data. Yet the specific skills that they use to do their work evolve rapidly.
“Digital technologies are constantly regenerating and transforming. We need to think about this ongoing transformation as a part of occupational identity,” says Vaast. “It doesn’t only change once, but repeatedly.”
Upgrading today for the future
For aspiring data scientists, that means that an education in data science is not an end in itself. Education is only the first step on a life-long learning journey where the ability to acquire new skills is the real key to success, both for individuals and the organizations they work for.
“Whatever the data scientists of the future do, there will not be a single certification,” says Vaast. “The competencies that are needed to get a job at the entry level are just the beginning; their skills will need to be updated throughout their career.”
In an extremely competitive job market, data scientists who are most successful are those who constantly update their expertise.
“Some of this comes from formal study, and some of it comes from working with data sets and new research teams,” explains Vaast. “There are multiple ways to gain expertise, but you not only need to get that expertise, you need to maintain it. That is the tricky part.”
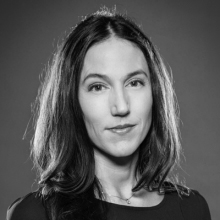