Artificial Intelligence innovation thrives in an environment where business arenas, fundamental research, and thought leadership overlap. As seen in recent cross-sector AI initiatives, determining the capacities of AI and related data analytics applications is essential to understanding how they will play out in the wider world, whether analyzing healthcare data or implementing predictive analytics in retail. On the Delve podcast, Desautels Professor Maxime Cohen demystifies how retailers can use data analytics to predict demand, make operational decisions, and boost revenue.
Subscribe:
“It’s impossible for the human brain to process that much information and to find all the hidden patterns and correlations between different types of features in order to make accurate predictions,” says Cohen. “That’s why in the specific case of demand prediction in retail, machine learning algorithms are very useful and have been successfully applied to get very high prediction demands.”
Demand prediction is part of broader data-driven strategies that give retailers the tools to more easily track and analyze their businesses and react to changes in real-time. With information gathered from inventory, suppliers, distributors, points of sale, consumer feedback, and many other sources, the retail industry has never wanted for data, which has grown massively through the expansion of online spaces. Retail organizations’ biggest challenge today is in using data analysis as an accurate tool for growth.
Why use predictive data analytics?
In his recent book, Demand Prediction in Retail – A Practical Guide to Leverage Data and Predictive Analytics, published by Springer this year, Cohen and his co-authors (Paul-Emile Gras, Arthur Pentecoste and Renyu Zhang) provide a detailed guide for data scientists and students of business analytics. The book offers business insights to a wider audience as well, pointing to something essential for all retailers today: how to leverage their historical data to predict future demand for their products.
Across verticals, from small fashion retailers to large electronics corporations to supermarkets, data analytics can be leveraged to inform and improve operational decisions. “The goal is to capture data from every single key stakeholder of the company,” says Cohen. “You really want to get a 360 view of your operations through a data lens.”
While Cohen’s book delves deeply into the technical details for data scientists, its focus is on retailers who want to embrace more data-driven practices. Each chapter illustrates each step in the demand prediction process, from data collection to pattern visualization to evaluating the demand prediction accuracy. The book and its accompanying website also provide a dataset and snippets of free programming code that can be replicated as needed.
“Readers can potentially use their own data from their own store to predict the demand,” says Cohen, adding that the book’s conclusion includes tools to use for heading into the prescriptive analytics realm. “We wanted to provide that to try to democratize access to this type of knowledge, because it can really help a lot of retailers.”
Leveling up with analytics
How to get a 360-degree overview requires strategic planning with data. In data analytics, including AI analytics, there are three levels of maturity at the moment, Cohen explains, though many organizations are only now reaching beyond the first level.
- Level 1: Descriptive analytics or descriptive AI answers the question of what happened in the past, with the goal of understanding key patterns and trends and identifying customer segments, for example.
- Level 2: Predictive analytics or predictive AI answers the question of what will happen in the future, with the goal of predicting demand for specific products at specific prices.
- Level 3: Prescriptive analytics or prescriptive AI answers the question of how to make optimal decisions in the future, with the goal of analytics being able to make recommendations for organizations to based decisions and actions on.
Demand prediction for decision making
Though just five years ago it wasn’t common for retailers to use predictive analytics, the COVID-19 pandemic issued a wakeup call to collect more data, find ways to monetize this data, and use it to improve operational decisions, says Cohen.
“It’s very important to nail down predictive analytics before moving to prescriptive, because any prescriptive will first need to have good predictive foundations,” Cohen explains. “It’s going to highly depend on the company and the appetite of the company to become more data driven and technology driven.”
Being able to predict demand based on accurate data analysis can guide several tactical and strategic decisions. In inventory management, businesses can better manage their inventory and replenishment strategy when they know exactly how much to purchase and to maintain in the warehouse.
“In that case, you’re going to have the exact level of inventory that you need, not one extra and not one less. You’re going to have no stockouts. You can reduce waste and increase customers loyalty and satisfaction,” says Cohen.
Predicting demand can help guide decisions not only on inventory, but on supply chain, pricing and promotion, procurements, fulfillment, and other areas. “You need to ask yourself, what are the data that are somewhat correlated or that are helping me to inform demand information,” Cohen illustrates. “You can list all the data sources, collect as many as possible, and then join all those data sources in order to predict the demand.”
Moving beyond data challenges
Though this process may appear straightforward when the code and purpose are clear, there are still challenges to demand prediction. “First of all, there was a cultural change,” says Cohen. “Convincing managers who have done their job for many years to trust a machine or adopt an algorithm to do those type of jobs that were done by humans is a big challenge in terms of change management.”
The other major challenge is in recruiting data scientists and building data infrastructure, which requires funds not only to build but to protect. “That’s why it’s not yet democratized the way I wish it were,” says Cohen. “I hope in the future, it’s going to become more and more prevalent.”
For more insights, listen to the full interview with Professor Maxime Cohen on the Delve podcast.
This episode of the Delve podcast is produced by Delve and Robyn Fadden. Original music by Saku Mantere.
Delve is the official thought leadership platform of McGill University’s Desautels Faculty of Management. Subscribe to the Delve podcast on all major podcast platforms, including Apple podcasts and Spotify, and follow Delve on LinkedIn, Facebook, Twitter, Instagram, and YouTube.
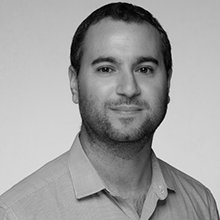