To eliminate discrimination in AI, algorithms should see race and gender, according to new research.
Amazon employs hundreds of thousands of people, and when you are building a workforce that’s the size of a medium-sized city, hiring top talent is a gargantuan task. Artificial intelligence holds promise to make human resources more efficient. But when the Seattle-based e-commerce behemoth implemented a machine learning algorithm to identify talent, that algorithm created an unforeseen issue.
“Algorithms like Amazon’s use historical data from existing employees to identify patterns between their characteristics and qualifications, and use these patterns to predict the suitability of job applicants,” says Warut Khern-am-nuai, an assistant professor of information systems at McGill University’s Desautels Faculty of Management.
“They try to use applicants’ characteristics in their CVs to predict qualification. The problem is that it is very possible that there has been some discrimination in the past. For example, most tech companies have historically hired more male candidates than female, and more white candidates than visible minorities. Because of this, machine learning algorithms will predict that applicants from the majority group, white male candidates, are more likely to be qualified for the job, while female or visible minority candidates who are otherwise similar are less likely to be qualified for the job according to the prediction.”
Amazon realized that its algorithm was biased against women and, in 2018, it got scrapped. But machine learning algorithms continue to hold great potential for automating talent management in large companies. They just need to be fair.
Approaches to algorithmic fairness modify outcomes, but overlook long-term consequences
To make algorithms “fair,” some computer scientists have made them “colour blind.” The core idea behind this type of algorithm is to completely remove sensitive variables such as race and gender from consideration. However, it turns out that such an approach does not necessarily prevent discrimination. A colour-blind algorithm might, nevertheless, interpret attributes that are correlated with race or gender in negative ways—as Amazon’s algorithm did.
Another way that computer scientists have sought to achieve fairness is through the demographic parity fairness constraint. This approach treats characteristics like race and gender as protected classes within the algorithm and selects candidates from these protected classes at the same rate that they do for unprotected classes, such as white men. Yet this approach introduces an entirely different set of issues, according to Khern-am-nuai.
“This type of algorithm manipulates prediction results so that male and female candidates are accepted at the same rate, which is essentially the same as affirmative action,” says Khern-am-nuai.
“When we use this technique, we are not modifying the prediction process. We are modifying the results. Some believe that as long as the algorithms predict male and female candidates at the same rate, they are fair; but by modifying the result, you are also changing the criteria for inclusion.”
Those favoured by algorithms could have less incentive to pursue additional credentials
Khern-am-nuai argues that changing the threshold for selection by a hiring algorithm means that candidates from some groups will be identified as suitable with lower levels of credentials such as education or achievement. Over time, those who don’t need the extra training to land the job will recognize this and could choose not to pursue additional education or training, to their own detriment.
“If an algorithm outputs male and female at the same rate, even though the threshold is different, female candidates will recognize that the output is biased in their favor. As a result, the use of this type of ‘fair’ algorithm will give them less incentive to be as qualified for a job.”
In practice, this might result in women needing less credentials or lower-standard performance reviews to meet an algorithm’s thresholds for inclusion. In the near term, women could benefit by obtaining employment more quickly after graduation, finding work with highly desirable employers or progressing into middle management positions more rapidly. But it could also stunt their career prospects in the long run because they might be hired to fill a position for which they are under qualified or ill-suited.
Algorithms could be made fairer by actively suppressing the ways that discrimination impacts other variables
In a new study, Khern-am-nuai and co-authors Hajime Shimao (Santa Fe Institute), Junpei Komiyama (University of Tokyo), and Karthik Natarajan Kannan (Purdue University) propose a new approach that optimizes the way algorithms process data, without modifying the outcome.
Their method suppresses the relationships that the sensitive variable—such as race and gender—has with other variables that are being considered. This helps obtain predictions that are fairer and ensures that the prediction results are incentive compatible for prediction subjects.
“We want to suppress the variables that are related to the source of the bias,” says Khern-am-nuai.
“We want to suppress the variables that are related to the source of the bias,” says Khern-am-nuai.
For example, high school grades might be lower for students who attended schools in economically disadvantaged areas, which often have many Black students. But those same students could excel in their studies and make valuable contributions.
“A human resources professional might recognize that their high school grades are unlikely to impact their performance after graduating university, but an algorithm might decide that Black people or women are not suitable because a characteristic is not similar to the organization’s current employees.”
The approach aims to make algorithms better at recognizing talent from traditionally underrepresented groups—without having to widen the criteria for inclusion. It might not select as many candidates as demographic parity algorithms, but those it does select should be similarly qualified.
In this sense, artificial intelligence and human intelligence may not be so different after all. Both need to recognize the diverse impacts that discrimination on the basis of race and gender has had, in order to create more equitable outcomes.
There is also another reason to ensure that algorithms are fair—companies using algorithms that discriminate on the basis of race or gender could be vulnerable to lawsuits.
“If you are using an algorithm to hire people, and it is biased against an identifiable group of people, that could be illegal. In the United States, it is against the Civil Rights Act of 1964.”
Khern-am-nuai further argues that ensuring algorithms are fair is not simply a matter of justice, legality, or ethics; it is also good business.
“Algorithmic bias could mean that you lose some good candidates—and often these will be candidates from a minority group. If you want to make sure that the highest quality candidates are fairly considered, the strategic best-response fairness algorithm is aligned with that objective,” he says.
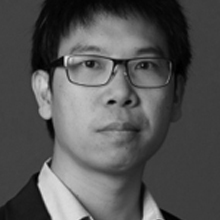