Discover how technology is changing the way people work and organize in an unprecedented way—and how we can adapt
New technologies have always given way to greater automation and increases in productivity, as well as the loss of jobs and the creation of entirely new ones. As any history buff would confirm, these transformations are to be expected.
But to say that learning algorithms—the latest technological feat—are just another chapter in humankind’s long history of innovation and adaptation would be misleading.
When it comes to altering jobs and the way people organize, learning algorithms (i.e. technologies that build on machine learning, computation, and big data statistical techniques) have unprecedented consequences, says Samer Faraj, Professor of Management and Healthcare at McGill University.
In new research, Faraj and his co-authors, Stella Pachidi (University of Cambridge) and Karla Sayegh (PhD student, McGill University), identify key aspects that make learning algorithms uniquely consequential in the context of work and argue that their role in the workplace will be determined by how we respond to their implementation.
What sets algorithms apart?
The power of learning algorithms lies in their ability to plow through an immense quantity and breadth of information to identify patterns. “As a result, we are seeing the regime of quantification take hold in many fields, from journalism and law to the management of human resources, and the implications are profound,” says Faraj.
Now more than ever, virtually every dimension of human life is tracked and quantified.
Now more than ever, virtually every dimension of human life is tracked and quantified. And in the context of work, we risk reducing employees to a set of measurable dimensions and making predictive judgments based on the likelihood of action, rather than on actions themselves.
For example, in select U.S. states, algorithms are used to decide whether an inmate should be granted parole; in such cases, the algorithm renders a decision based on whether the inmate’s data correlates with the profile of a repeat offender.
“This raises a number of issues. If learning algorithms are relied upon for their supposed objectivity, we need to remember that they are political by design,” Faraj cautions. Whether implicitly or explicitly, they are imbued with the value choices of their creators, which are made in informal and intuitive ways.
Faraj also provides an example from the banking industry, where algorithms are used to determine if someone is a viable loan candidate. “The process that determines what constitutes a credit-worthy applicant will reflect the beliefs of the practitioner who pre-processes the data and pre-classifies the training dataset,” explains Faraj. “If the algorithm determines that there is a negative correlation between living in a low-income neighborhood and the likelihood of loan repayment, we could end up discriminating unjustly against minority applicants.”
Rethinking human learning
In a world where computers can evolve, humans will need to rethink the way they learn as well.
“Technological revolutions bring the redefinition of roles and the reorganizing of the workforce,” says Faraj. “With the introduction of internet search, for example, librarians had to adapt; they repurposed their expertise and re-conceptualized their professional identities.”
We are seeing a similar situation with radiologists who spend their careers training to identify problems on scans. In recent years, image recognition software has improved to the point where computers are as accurate as (or better than) radiologists in identifying abnormalities on a medical scan.
On one hand, the technology frees radiologists from the rote task of identifying everyday problems to focus on more challenging cases, thus further developing their expertise.
On the other hand, those rote tasks are important training mechanisms that help people become experts in the first place. Without the repetition of seeing 100 mundane scans, how can a radiologist learn to identify an abnormal one?
“Our models of learning are changing,” Faraj says. “We typically start at the bottom, do the mundane, and graduate slowly to more complex cases. There’s now a big question mark about how we train people for a future where computers can learn better than we can.”
The ideal future he sees is one where AI is used to help humans learn better and adapt more quickly. He points to some schools in China, which have implemented artificial intelligence programs in classrooms; the software creates personalized curriculums based on each child’s needs and pace of learning, and the teacher remains in the classroom to help as needed.
While learning algorithms may force us to adapt and re-learn our roles, if we approach them correctly, they might also fundamentally improve the way that we absorb information.
People are not machines
One of the biggest risks of algorithm learning is the devaluation of the human workforce. “Learning algorithms have given rise to a tension because they threaten the legitimacy of professional expertise,” Faraj explains. While learning algorithms yield greater business efficiencies, it is also important that we not reduce workers to mere datasets.
Today Amazon warehouse employees are rigidly tracked as they find and deliver goods in mammoth facilities, sales associates are judged on their ability to make targets, and journalists are rewarded when their stories perform well online. The result is an over-burdened workforce that may not be focused on the right metrics. In the case of journalists, this might result in focusing on click-baiting sensationalism rather than on genuine insights; for sales associates, it might mean closing deals at any cost.
A major risk to employers is the resultant loss of talent. “Reducing management to an algorithm would be counter-productive in the long run,” Faraj says. “Rather than relying on algorithms to maintain employee accountability, companies should use humans to ensure that learning algorithms remain accountable.”
This can (and should) come from the top, but that doesn’t mean there aren’t also opportunities for the labour force to mobilize and keep the algorithms that shape their daily lives accountable. For example, journalists can lobby to monitor success based on qualified reader engagement (i.e. did they read to the end of the article or share it on social media?) over a single page view.
As algorithms become more sophisticated and ubiquitous, we also need to ensure that we are leaving room for creativity. Faraj cites the example of Slack, a workplace communication application that scans a user’s unread messages and flags those deemed most relevant by its algorithm. “While this might enhance coordination at work, the algorithm is simultaneously limiting the information that employees are exposed to, which can hamper knowledge diversity and organizational innovation,” explains Faraj.
In the age of the learning algorithm, the line between distinctly human and machine competencies has blurred, leaving many uneasy about how this interplay will evolve.
“More than with previous technological change moments, we will need to implement the right policies to regulate the designer goals behind the development of such highly performative technologies,” explains Faraj. In the end, he says, it’s important to see these programs for what they are—tools: “Learning algorithms should empower the workforce, not replace them.”
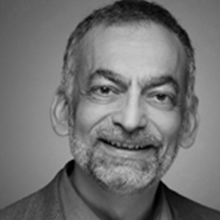