Despite its reputation as a disruptive technology, AI does not handle disruption well
- COVID-19 has disrupted our behaviours, playing havoc with AI systems.
- Subject matter experts who understand the context in which an AI system is operating should assess how the COVID-19 crisis may have affected it.
MIT Technology Review reported last week that a number of AI systems are breaking down in the context of the COVID-19 pandemic. Systems designed for tasks ranging from inventory management to recommending online content are no longer working as they should.
The impact of the coronavirus crisis reflects a well-known limitation of AI systems: they do not handle novel situations well. Indeed, two months ago I warned of the challenge of using AI during the COVID-19 crisis. At the time I focused on the difficulty of creating new algorithms to address COVID-19 problems, but this same issue also poses problems for pre-existing AI systems.
Now is the time, therefore, to revisit the AI systems currently deployed in your own organization and assess whether they are still viable in this COVID-19 era.
Why is AI having problems?
Almost all current AI systems use some version of machine learning, which work by examining large amounts of training data, usually past examples of the task it is trying to learn (e.g. past examples of inventory fluctuations, or content streaming behaviour). Machine learning systems look for patterns in the training data, and then once deployed, they draw on those patterns to make predictions for new cases (e.g. what will inventory needs be next week, what will a given new user enjoy).
To learn completely new rules, machine learning algorithms need large amounts of new dataThe machine learning approach works well when these new cases are similar to the examples in the training data. The ability of machine learning algorithms to identify subtle patterns in the training data can allow it to make a faster and possibly better prediction than a human. However, if the new cases are radically different from the training data, and especially if we are playing by a whole new rulebook, then the patterns in the training data will no longer be a useful basis for prediction. Some algorithms are designed to continuously add new training data and therefore update the algorithm, but with large changes this gradual updating will not be sufficient. To learn completely new rules, machine learning algorithms need large amounts of new data.
In addition to lacking relevant examples in the training data, AI systems may falter if new factors become important that were not considered in the original design. AI developers select which pieces of information to include in the training data, anticipating all of the factors relevant to the task. Radical disruptions such as the COVID-19 pandemic may mean that completely new factors that are not even part of the algorithm suddenly become important. Such situations will require redesigning the system itself, ranging from a small tweak to a complete overhaul.
Anticipating AI problems
Not all AI algorithms will face performance problems under COVID-19. Given that, you could simply hope that your system will be unaffected or monitor it for declines in performance. A more proactive approach, though, is to anticipate problems and take strategic action to address them. Anticipating problems does not necessarily require understanding the technical aspects of AI. Indeed, more important is subject matter expertise, someone who understands the context in which the AI system is operating and how the COVID-19 crisis has affected it.
The subject matter expert should examine two aspects of the AI system. First, what is the source of the training data? To what extent is that training data still relevant today? Are current situations similar enough to the examples in the training data that the patterns that the algorithm has identified are still relevant?
Second, what factors does the system consider and what assumptions about cause and effect are baked into its design? Are there new factors influencing behaviors today that are missing from the system?
Developing an action plan
If your analysis suggests that the COVID-19 pandemic may cause problems for your AI system, you have a few options.
We humans are also struggling to understand how the world works in this COVID-19 era, but we are better-equipped to deal with novel situations in most cases than AIIn the short term, it will likely be necessary to increase human oversight. We humans are also struggling to understand how the world works in this COVID-19 era, but we are better-equipped to deal with novel situations in most cases than AI. It may even be necessary for humans to take a leading role for a while. For example, Facebook is using fact-checking organizations to initially identify cases of COVID-19 misinformation, and then pairing these with an AI system to flag the many variations of each of these cases that would otherwise overwhelm fact-checking capacities. As the AI system is provided with more examples of COVID-19 misinformation, it will learn patterns and increasingly be able recognize new cases on its own.
Similarly, if the lack of relevant training data is the central problem for your system, you can seek to collect new training data that fits current conditions. If you have identified newly relevant factors that are not currently considered in the model, then you would also need to redesign your AI systems to take these factors into account.
These steps, though, may not be worthwhile if you expect the context to change radically again. In the case of identifying fake news, we might reasonably expect that COVID-19 misinformation will, going forward, evolve slowly enough that training an AI system to detect it would be worthwhile. The important impact of this misinformation as well as the large volume of data that is already being generated also make such a redesign worthwhile. In contrast, it is not clear that the abrupt consumption changes that have affected inventory AI systems represent a stable “new normal” that is worth the time and expense to model.
The larger lesson
Despite its reputation as a disruptive technology, AI does not handle disruption well. While the COVID-19 pandemic has highlighted this problem, it is an ongoing weakness. Even slow changes can accumulate to significant drift that threatens AI systems. Regular monitoring and revisiting, therefore, is necessary. Rather than getting lost in the technical details, though, the most important step in monitoring an AI system is understanding the changing landscape. Ensure that the system still fits that landscape, whether it is evolving slowly or shifting with disturbing speed under our feet.
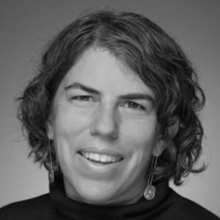