Artificial Intelligence has solved many problems in our increasingly data-oriented world, and as AI technologies improve, they’re set to increase accuracy, efficiency, and knowledge across all sectors. But in today’s reality, the success of AI depends partly on its application. In the interdisciplinary arena of management, AI is being applied to big-picture issues of organizational strategy and supply chain operations, as well as the ethical details of human resources and the many moving parts of the retail industry.
Over the past two years, Delve has shared management research on how the COVID-19 pandemic shed new light on global issues, from healthcare systems to climate change. The world of work also continues to absorb the pandemic’s impact, whether in supply chain management, global financial shockwaves, or human resources. Meanwhile, new AI technologies are providing solutions to novel problems that cross disciplines and sectors.
How can Artificial Intelligence, including new machine learning algorithms and consumer-level data analysis tools, augment and even improve management of and within organizations? Researchers at the McGill Desautels Faculty of Management provide insights on how AI can be used to increase organizations’ knowledge and operations through data analysis and even contribute to strategic organizational change.
Three recent Delve podcast episodes and accompanying articles tackle the role of AI in different management arenas, diving into:
- How a real-time AI model can provide insights on panic buying and other changes to consumer purchasing.
- A new toolkit that aims to ensure ethical and responsible use of AI tools by Human Resources professionals.
- How using machine learning algorithms for data analysis in retail can increase the accuracy of forecasting product demand.
New Normal: How AI Is Reshaping Post-Pandemic Retail with Warut Khern-am-nuai
Shifts in consumer spending are a constant in retail, but the first wave of the COVID-19 pandemic in the spring of 2020 induced an unexpected level panic buying, sending groceries, toilet paper, and more unexpected items flying off the shelves. How can the retail industry use consumer data to learn from these incidents and ward off a repeat situation?
In episode 6 of the second season of The “New Normal” podcast series with host Dave Kaufman, Desautels Professor Warut Khern-am-nuai discusses the data behind pandemic buying and how retailers can use an Artificial Intelligence framework to react in real time to unexpected fluctuations in consumer purchasing.
“If we were to develop the predictive model to predict panic-buying behaviour, it’s not going to work as well as intended,” says Khern-am-nuai. “Instead of trying to predict panic-buying behaviour, we detect the panic-buying behaviour pattern in real time. When it occurs at any store in Canada, we can detect that as soon as five minutes after it happens.”
Khern-am-nuai expands on his earlier research on pandemic panic buying to examine the retail industry throughout the COVID-19 pandemic and look to the future of AI in retail, including how one small policy change by retailers could have avoided empty shelves in the first place. “If we had this tool before the first wave of panic-buying behaviour, and if stores applied this tool, then they could have improved the access to essential goods by approximately 56 percent,” he explains.
An Ethical, Human-Centred Approach to AI in Human Resources, with Matissa Hollister
Hundreds of AI-based tools already exist for use in Human Resources tasks, including hiring, training, and employee engagement, but it’s often difficult to discern their use value, let alone how to use them effectively and ethically, an arguable essential in HR. How can organizations navigate the responsibilities and challenges of implementing Artificial Intelligence in Human Resources?
On the Delve podcast with host Robyn Fadden, Desautels Professor Matissa Hollister discusses how organizations can navigate and overcome the responsibilities and challenges they face when implementing AI in Human Resources.
“AI is a cutting-edge tool that encodes the status quo,” says Hollister. Since AI learns from existing real-world data, all the world’s aspects—including bias, unfairness, and inequality—end up being reflected in the data and can be encoded in the system. “The system is not capable of discerning what aspects of the real world we like and we think should be kept, versus the aspects of the real world that we’re not so happy about and we would like to get rid of.”
In collaboration with the World Economic Forum and over 50 other researchers, industry experts, and HR professionals, Hollister recently authored the toolkit and accompanying white paper Human-Centred Artificial Intelligence for Human Resources: A Toolkit for Human Resources Professionals. The toolkit is up-to-date guide on key topics and steps in the responsible use of AI-based Human Resources tools, and includes checklists focused on strategic planning and the adoption of specific tools.
How Predictive Data Analysis Illuminates the Future of Retail, with Maxime Cohen
Cross-sector AI initiatives, where academic research addresses business and management needs, have shown that determining the capacities of AI and related data analytics applications is essential to understanding their real-world functionality. How can retail businesses use data analysis to predict future demand for their products?
On the Delve podcast with host Robyn Fadden, Desautels Professor Maxime Cohen demystifies how retailers can use data analytics to predict demand, make operational decisions, and boost revenue. In his recent book, Demand Prediction in Retail – A Practical Guide to Leverage Data and Predictive Analytics, Cohen and his co-authors provide a detailed guide and business-related insights for data scientists, students of business analytics, and retailers in general on how to leverage past retail data to predict future demand for products.
“It’s impossible for the human brain to process that much information and to find all the hidden patterns and correlations between different types of features in order to make accurate predictions,” says Cohen. “That’s why in the specific case of demand prediction in retail, machine learning algorithms are very useful and have been successfully applied to get very high prediction demands.”
Delve, the official thought leadership platform of McGill University’s Desautels Faculty of Management, covers all areas of research at Desautels. Delve’s podcast is hosted by Robyn Fadden, while Delve’s The “New Normal” podcast series is a collaboration between journalist Dave Kaufman and . The “New Normal” is produced by Delve and Dave Kaufman, with audio engineering by David Rawalia. Each episode looks in-depth at a different aspect of the new normal that we are all navigating due to the COVID-19 pandemic. Original music by Saku Mantere. Dave Kaufman is a Montreal-based journalist and commentator. He has worked for CJAD 800 and TSN 690 Radio in Montreal, CTV News Channel, CTV Montreal, and TalkRadio and SkyNews in the United Kingdom. He has written for the National Post, Montreal Gazette, and Toronto Sun and other publications. Follow him on Twitter at @TheKaufmanShow.
You can subscribe to the Delve podcast on all major podcast platforms, including Apple podcasts and Spotify, and follow Delve on:
LinkedIn, Facebook, Twitter, Instagram, and YouTube.
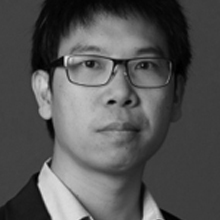
Warut Khern-am-nuai
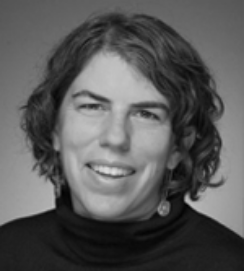
Matissa Hollister
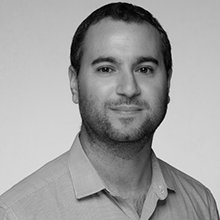